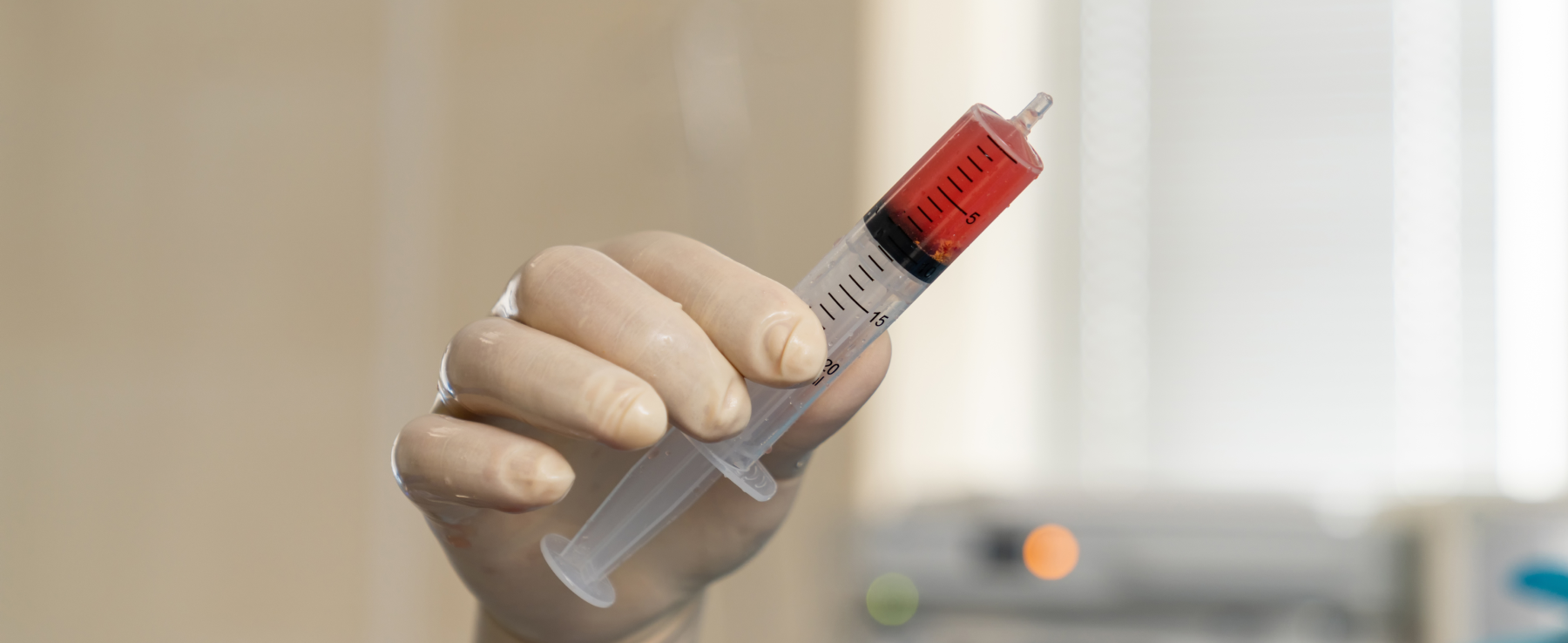
Predicting Graft vs. Host Disease
A risk assessment tool for critical side effects of bone marrow transplants.
Partner
Dr. C. Strahlendorf
BC Children's Hospital
The Digital Lab
Status
Upcoming
technology
Overview
Context
Blood-related cancers like leukemia often require bone marrow transplantation during the course of treatment. This life-saving treatment comes with a life-threatening risk: Graft-versus-host disease (GvHD). GvHD occurs when the donor bone marrow recognizes the host as an invader and attack the patient.
Despite significant advancements, this side-effect is the leading cause of long-term morbidity and non-relapse mortality after bone marrow transplantation. Its incidence among children is estimated between 40 and 80% for acute GvHD, and 6 to 33% for chronic GvHD. The severity of each case varies, and it does not occur in every patient. Moreover, GvHD is relatively understudied in children. Understanding and predicting which patients are at risk of developing GvHD is crucial to planning treatment and preventative care after transplantation.
In this study, we are exploring the potential risk factors for GvHD from a data-driven approach. Our aim is to use these risk factors alongside machine learning models to predict both the risk and severity of GvHD occurring and compare the performance of our models against gold standard methods.
Process
Graph vs. Host Disease (GvHD) does not appear consistrently and varies in severity. With every patient having unique characteristics, it is difficult to know who may be at risk of this potentailly life-threatening side-effect. Any amount of these characteristics could determine their risk, but the patterns are often very complex with significant amounts of data to sift through. Using data science techniques like dimensonality rediction and machine learning algorithms, we are developing a model that can predict the risk of GvHD developing and its severity.
A key consideration in this project is determining what data will be available at the time when an accurate prediction would be most useful. This presents a tradeoff: while more data can improve predictions, if it is only available after transplantation or other critical treatment stages, the prediction may be less valuable to clinical teams. To address this, we are working closely with clinicians to identify readily available information and ensure this tool is practical and impactful in a clinical setting.
Funding information
Publications & Resources
Potential Impact
Predicting the occurrence and severity of GvHD has twofold benefit. The primary benefit is that for an individual patient, treatment planning and interventions can be informed and directed with their risk profile for developing GvHD in mind. The earlier a prediction is generated, the earlier care can be planned to minimize this risk and improve patient's outcomes.
Alongside bettering patient care at BC Children's Hospital, understanding how models predict GvHD may improve our understanding of the disease itself among pediatric populations.